It’s a topic many in the IT / Cyber security world have been talking about for a long time – the topic of online anonymity.
In this blog post, I take a look at a recent study by Researchers at the North Carolina State University Raleigh which uses a collective of data to identify the home locations of Strava users who have enabled the anonymity function in a misguided attempt to hide where the live.
Staying anonymous is hard to do
Most people are aware of the reasons to keep certain data hidden from others, yet we live in a world where living online requires us to regularly expose much of that data.
In a singular view, a piece of data may be fairly anonymous, but online, that data is regularly augmented with millions of other data to create relationships and trends – most of the time by organisations who wish to sell us things, but in some cases it is by law enforcement and intelligence services, and in other cases by criminals who want to use our data to commit acts of fraud or theft.
Application and service providers inform us that our data is safe and anonymised whilst in their hands, but is that really true?
Well – like I said earlier – in singular forms yes, it’s mostly true, but when aggregated with all the other readily available data sources, that anonymity is quickly removed.
Strava heatmaps
Strava is an app I’ve blogged about previously which allows users to share their activity routes (cycling, running, walking, etc.) online. It has come under criticism in the past in that it could allow others to misuse this data, so back in 2018, the designers added an anonymity feature that allows users to block out sensitive areas from their routes – such as home locations.
However – this in itself adds more data to the ever-growing pile and in that earlier post, I wrote about how researchers had managed to triangulate users map visibility zones to work out probably home locations.
Now, researchers at Carolina State University Raleigh have taken the next step and used other data to pin-point Strava users locations, making the whole anonymity function near-useless.
Assistant Professor Anupam Das, Undergraduate researcher Kevin Childs, student Daniel Nolting released their paper “Heat marks the spot: De-anonymizing Users’ Geographical Data on the Strava Heatmap“
How they did it
The first thing the researchers did was to collect publicly available data from Strava heatmaps over a period of a month for the states of Arkansas, Ohio, and North Carolina.
Next, they used image analysis to detect start/stop areas which were next to streets, indicating that a specific home is linked to a source of tracked activity.
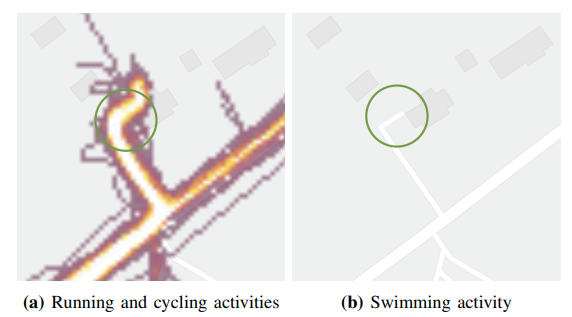
After selecting a number of possible candidate data, the researchers aligned the Strava data with imagery from OpenStreetMap to identify individual residence locations.
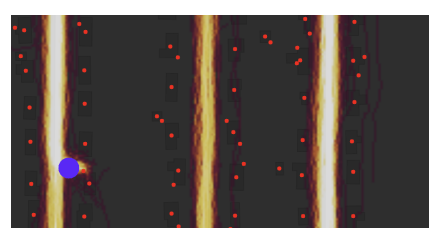
To de-anonymise users, the researchers then used a feature in Strava which allows users to search for other users by name or by city. Searching by city lists all users in that location who have set the city in their profile.
After retrieving lists of users for cities matching their target locations, the researchers then turned to voting registration data for a matching name.
Inference analysis
Using their aggregated data, the researchers were able to identify home locations for registered voters who matched “anonymised” Strava data, thus de-anonymising said data and creating an inference that a specific Strava user lived at a specific address.
The researchers fully acknowledge that many Strava users do not start or finish their activites at a home location, instead they may drive to a rural location to start their run or ride, or may partake in sporting events at arenas, etc. so theis inference cannot be used to identify all strava users, but they say that thae system they used was accurate in identifying nearly 40% of users who started activities from home locations.
Possible issues with such information
In their report, the researchers posited a few scenarios where an attacker could use such de-anonymised data to locate an individuals home, not the dates/times/frequency a Strava user goes out and use this data to burgle their home. It’s alos possible that a user who follows a regular pattern for their activity could be attacked during said activity if an attacker could identify who the user is, and the regular route taken.
Of course these are worst-case scenarios, and are highly unlikely to ever happen, but it just goes to show that the security, safety, and anonymity features we take for granted with services such as Strava, aren’t necessarily as good as what we think they are.
Always be cautious about sharing personal data online, even if you think its anonymous – there are thousands of ways to de-anonymise information to build a picture of someone or something.